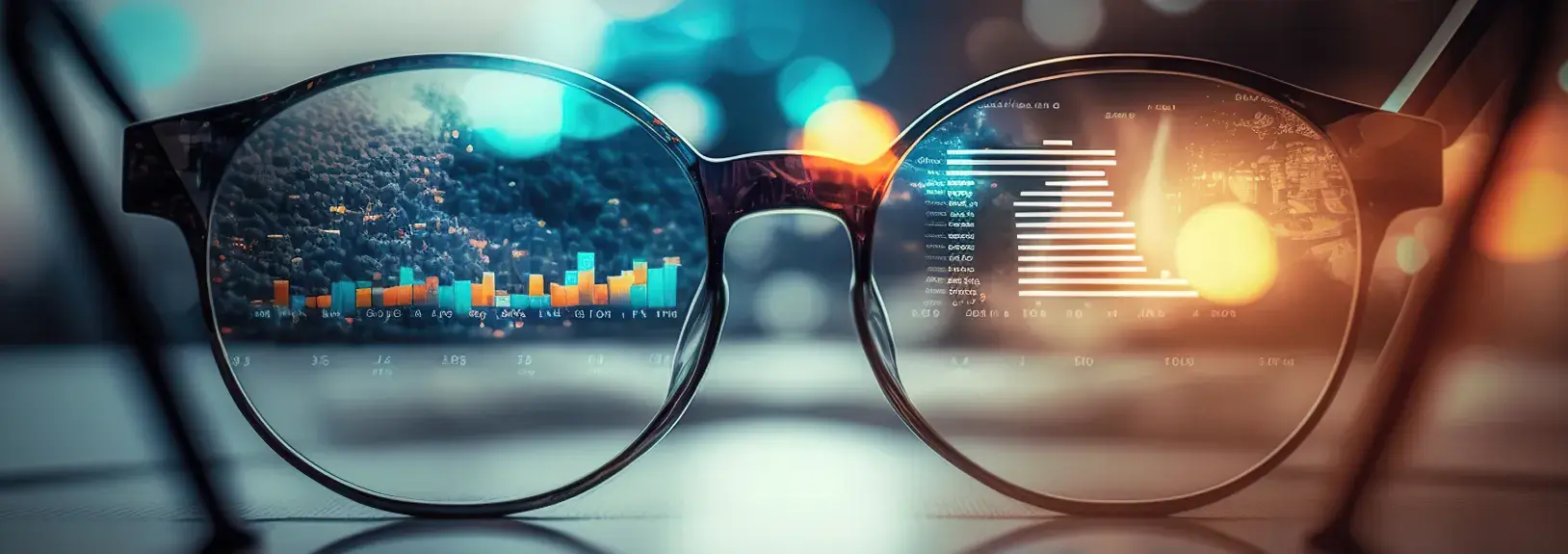
Study of the adaptive-ridge algorithm with applications to time to event data
Olivier Bouaziz (MAP5, Université Paris-Cité)
Résumé : The adaptive-ridge (AR) algorithm is an iterative method that was introduced as a penalisation technique designed to ensure variable selection and regularisation. This algorithm depends on two parameters, q (with 0 <= q < 2) and \delta (with \delta>=0). In this talk, I will show that:
- when 0 < q < 2, delta >= 0, this algorithm solves the minimisation of a (possibly non-convex) l_q penalised contrast
- when q=0, delta>0, this algorithm solves the minimisation of a squared-log penalised contrast which approximates the l_0 penalty when delta is “small”.
Different proofs exist for those results but I will focus on the Majorized-Minimized (MM) approach which can be derived from a simple variational reformulation of the l_q penalty.
In a second part of this talk I will illustrate the interest of this algorithm on some applications in survival analysis. In particular I will use the AR algorithm in the piecewise constant hazard model where, starting from a large grid, the number and locations of the cuts of the hazard function can be automatically determined with the AR algorithm by penalising on similar adjacent hazard values.
Reference: “A Review on the Adaptive-Ridge Algorithm with several extensions”. R. Abergel, O. Bouaziz, G. Nuel.
https://hal.science/hal-04051929/document